AI in Schools: Sal Khan’s Bold Predictions and What’s Still Missing
A deep dive into Sal Khan’s new book, plus more AI news, tools, and policy
Book Review: Sal Khan’s Brave New Words
Salman (Sal) Khan, founder of Khan Academy, is a leading thinker and doer when it comes to AI in education, so I was eager to read his new book Brave New Words: How AI Will Revolutionize Education (and Why That’s a Good Thing) for many reasons. Chief among them is the fact that Khanmigo, Khan Academy’s AI-powered tutor, is one of the most respected and widely used AI tutors. Kristen DiCerbo, Chief Academic Officer at Khan Academy, also participated in our recent gathering of education leaders where we discussed the “wicked opportunities” AI could offer in K-12 education.
Bottom line: I recommend the book! Brave New Words is an easy read with compelling ideas and concrete examples drawn from the early implementation of Khanmigo. Khan envisions AI as a coach and advisor to students, helping them not only push their own thinking on the material they are being taught but also navigate the internet more efficiently and cautiously. Khan sees a world where AI also acts as a parenting coach and makes parents better advocates for their students via vastly improved transparency about what and how their children are learning. This, I believe, is truly one of the biggest opportunities—and underexplored use cases—around AI.
Khan has a compelling vision for what Gen AI could do for K-12 education. He makes a strong case that teachers should not fear AI, but embrace it, and the book should be required reading in schools of education, if only as fodder for discussion and debate among teachers in training. Khan believes that AI will never replace teachers, but rather will be like a teacher’s aide in classrooms. In Khan’s view, AI should make the job of teaching more sustainable, push teachers to have higher expectations for students, and encourage teachers to focus on relationships and higher-order thinking instead of lectures and rote learning. The book goes deep into topics as wide-ranging as assessments, mental health, creativity, and the future of work. On each, he makes an engaging argument for how AI could accelerate better, richer instruction as well as greater opportunity for marginalized students.
But Khan’s vision is seen through rose-colored glasses. He does not address, for example, the issues that prevented similar innovations from taking hold under earlier tech-enabled models, like Rocketship Education. Rocketship was founded on John Danner’s ambitious plan for “Blended Learning” elementary schools that would scale up across the country. Students originally did 60-to-90 minutes of online learning every day in computer labs (using curriculum tools like Khan Academy) overseen by aides and rotated to different classrooms for math and literacy instruction. Before long, however, Rocketship abandoned the computer lab model (kids were acting out and not learning much online) and pivoted to a completely different instructional model that emphasized community and parent engagement.
Maybe Gen AI and next-generation models of ed tech like Khanmigo can overcome the Blended Learning failures of the past, but this case would be made much stronger by acknowledging past failures and providing evidence instead of assertions. Though Khanmigo is now in more than 50 school districts nationwide and educating roughly 65,000 students, the organization has yet to produce a study demonstrating efficacy or even showing how Khanmigo can be used most effectively. Nor has Khan Academy, for that matter (as far as I know).
Khan is very bullish on AI in education (as the title makes clear), and while his enthusiasm is exciting and infectious, it too often left me asking: “Where’s the evidence for that statement?” At one point, Khan breezily supports the idea of “flipped” classrooms where students engage with online lectures and other direct instruction at home, and classroom time is used to check for understanding and collaborate on projects. But what do we know about the outcomes of this instructional method, what level of teacher skill is needed to pull it off well, and do all students thrive with this model? Evidence to date suggests it can be problematic. The book also edged on self-promotion for Khan’s work in a discomfiting way. Several times, Khan makes assertions about the success of Khan Academy and Khanmigo without evidence to back up the claims. And while he often acknowledged the risks with AI and called for “guardrails,” he offered few specifics.
Despite my critiques of Brave New Words, I am a firm believer that it is critical, as Khan advocates, that we all “double down our efforts on using large language models for the good of society.” As Khan writes:
“The genie is out of the bottle, and the bad actors have the edge, but it is really a race. The countermeasure for every risk is not slowing down; it is ensuring that those favoring liberty and empowering humanity have better AI than those on the side of power and despotism.”
The “Mastery Versus Coverage” Dilemma
An interesting chapter on education (chapter nine, if you’re curious) in a new RAND report on Artificial Intelligence shows that some pedagogical and policy dilemmas will not be changed by Gen AI. In fact, some of the best personalized AI learning tools will encounter the same challenge that other instructional reforms have faced in the past: whether teachers should allow students to go deep on “mastery” or cover more “breadth” prepping for end-of-course exams. My research colleagues and I saw this dilemma firsthand in the Personalized Learning study CRPE conducted in 2018. At the time, teachers told us one of their greatest challenges was figuring out how to ensure that students who wanted to dive deep into a particular subject would not be left behind as the class moved on, due to pacing guides that dictated the teacher cover skills and knowledge at that grade level.
The title of the chapter says it all: “The Promise of AI to Transform Teaching Will Fail If School Systems Do Not Transform Too.” The essay argues that attending to policy questions (assessment, accountability, and incentives that drive pacing guides and whether teachers use end-of-course exams or mastery-based exams) will be critical if we hope to realize the potential of AI and prepare students for the AI workforce.
This also got my brain going on whether AI could help solve this dilemma in innovative ways. Mastery Public Schools in Philadelphia, a high-performing charter network, was designed to accelerate students who were many grade levels behind by providing different paths to graduation within the schools, some with far more intensive remediation supports. Could there be AI-powered school designs that personalize learning and still hit grade or grade-band level proficiencies? This could be a fun hack-a-thon.
What’s Next: AI Video Learning
From John Bailey: A cool new study on AI video.
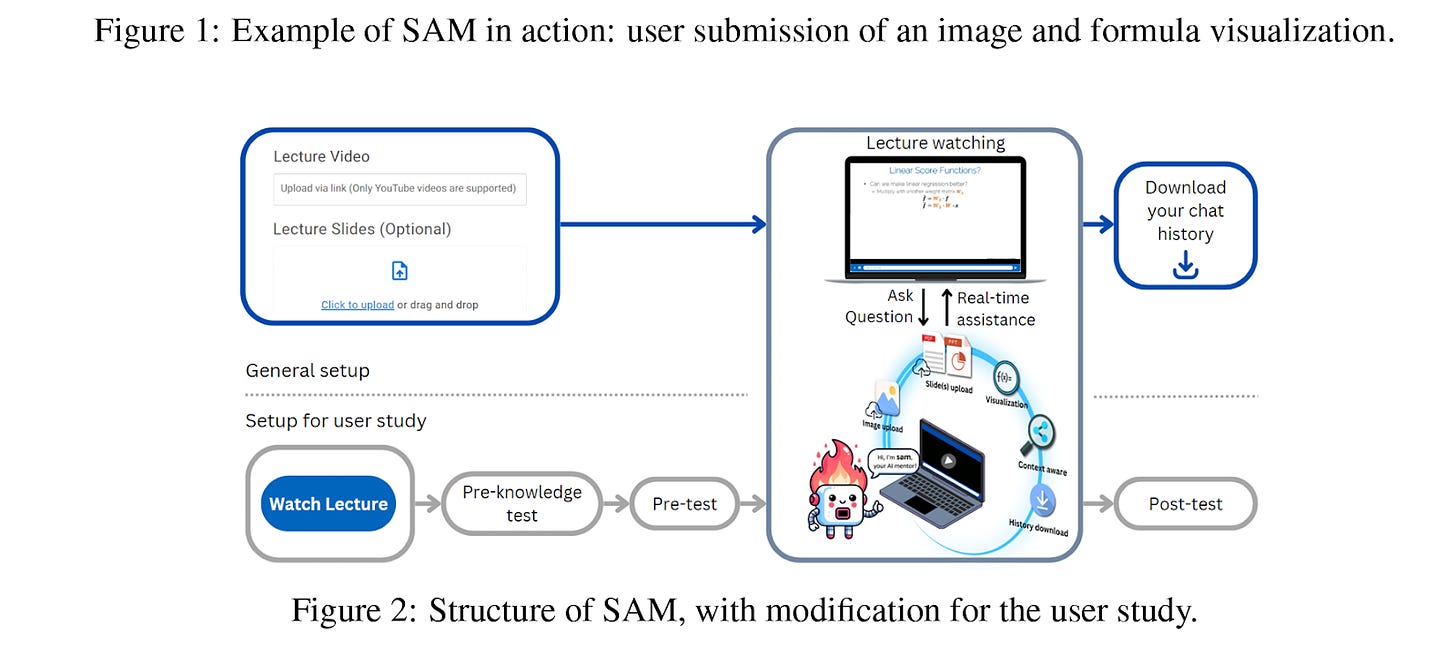
The results suggest that SAM represents a promising direction for interactive, AI-driven learning tools that foster student engagement and student ownership over education. Its context-aware assistance and real-time feedback were particularly effective for younger learners.
Along with rapidly developing AI video capabilities, new audio tools are out as well. Did you see my last post where I used Notebook LM to create a podcast from our recent State of the Student report? Google’s new Illuminate applies that very cool function to various research papers and select books. I love the idea. I listened to the six-minute podcast for Huckleberry Finn, one of my favorite books. Not bad, actually! It draws from current analyses and debates about the book, and talks about the themes, symbolism, characters, and relevance to current contexts. Not the most sophisticated analysis in the world, but far better than CliffsNotes!
Should AI Make Policy and Funding Decisions?
Um, no.
This recent New York Times story about Nevada’s recent attempt to use AI to identify at-risk students and allocate state funding has received a lot of attention. Basically, Nevada educators were not happy to learn that an AI-generated model decided that there were suddenly 200,000 fewer at-risk kids than before. The company that contracted with the state was less than transparent about how the “system” ended up identifying at-risk students, citing proprietary issues (which is going to be a perennial problem when education policy, politics, and AI intersect).
However, the real issue, it seems to me, is that state officials presumably gave the contractor a free license to decide on cut-off points for how much “at-risk-ness” warrants funding and other policy decisions. There may have been sound policy rationale behind the idea of putting more resources behind the most severely at-risk students (AI is just using data and logic, after all), but policy necessarily involves judgment and politics is always a factor in public education. Schools do not like to lose funding. Parents do not like to lose extra help for their children. Did state officials really think an AI-generated funding formula would come without significant pushback and controversy?? Lesson for states and other government agencies: AI can be a critical tool for analysis and informed policymaking, and the data and logic may open up important new considerations and challenges to the status quo, but there is no dodging human responsibility for policy, transparency, and politics.
Another cautionary policy tale: One district is being sued for allegedly unfairly punishing a student who was using AI for a classroom assignment. My colleague Bree Dusseault weighed in, noting that states are highly inconsistent in providing districts with guidance on AI policy.
Final Words
“This is not a drill. Generative AI is here to stay.”
Sal Khan, Brave New Words